Key takeaways
- Mortality projections from the CMI 2023 model for the cohort of UK residents currently aged 50-70 years old display worsening mortality for this group over the next several decades, especially for males.
- The negative mortality projection for males aged 50-70 appears to be driven by "deaths of despair" (drug overdoses, self-harm, etc.) concentrated in less affluent socioeconomic groups from the time when this cohort was aged 20-40.
- Projecting forward these earlier "deaths of despair” may overstate mortality rates and underestimate liabilities for this group of younger pensioners.
As actuaries and the others in the longevity community await additional updates to the UK Continuous Mortality Investigation (CMI) 2023 Mortality Projections Model (“CMI_2023”), it is a valuable exercise to revisit the models underlying CMI_2023 and critically analyze their appropriateness.
Of particular interest is the effect of the models when examining the mortality of UK residents currently aged 50-70 years. This 14-million-person cohort, the industry’s next massive group of retirees, arrives with unique mortality experience that may be negatively skewing mortality model outputs.
This analysis of that specific cohort is another effort by RGA actuaries to study the CMI model and share insights on its proposed calibration. (Earlier this year, RGA released a longer paper investigating the implications of the default calibration of the CMI_2023 version of the model.)
Background
Modeling expected future mortality is a complex topic. When building models, actuaries consider changes in healthcare provision and medical advances, changes in diet and lifestyle, and the impact of new technologies such as artificial intelligence, among many other factors.
One frequently used starting point is to fit statistical models to past data and extrapolate trends into the future. A common approach is to break down those trends into components, including:
- Factors that vary by age but are constant over time (age effects)
- Factors that vary by time but apply to all ages (period effects)
- Factors that apply to specific groups, or cohorts, of people throughout their lifetimes (cohort effects)
An example of this modeling approach
The industry mortality projection model in the UK (the CMI model) follows this approach. The model estimates age, period, and cohort effects from past data and projects them into the future. These effects are then separately blended into assumed long-term rates at different speeds, depending on age at the start of the projection period.
Of key interest here is that cohort effects can be projected forward up to 40 years for certain ages. Once actuaries estimate these separate projections, they add them together to estimate the change in mortality rate for each age in each calendar year.
The changes from year to year are typically referred to as “mortality improvements,” although the change could be negative, meaning mortality rates become worse (and mortality has therefore “dis-improved”). When dealing with a complex model like the CMI model, it is important to ensure the output is reasonable and can be justified from a qualitative point of view.
Investigating model output: Cohort effects in the UK
Figure 1: heatmap of improvements to mortality rates in the England and Wales general population
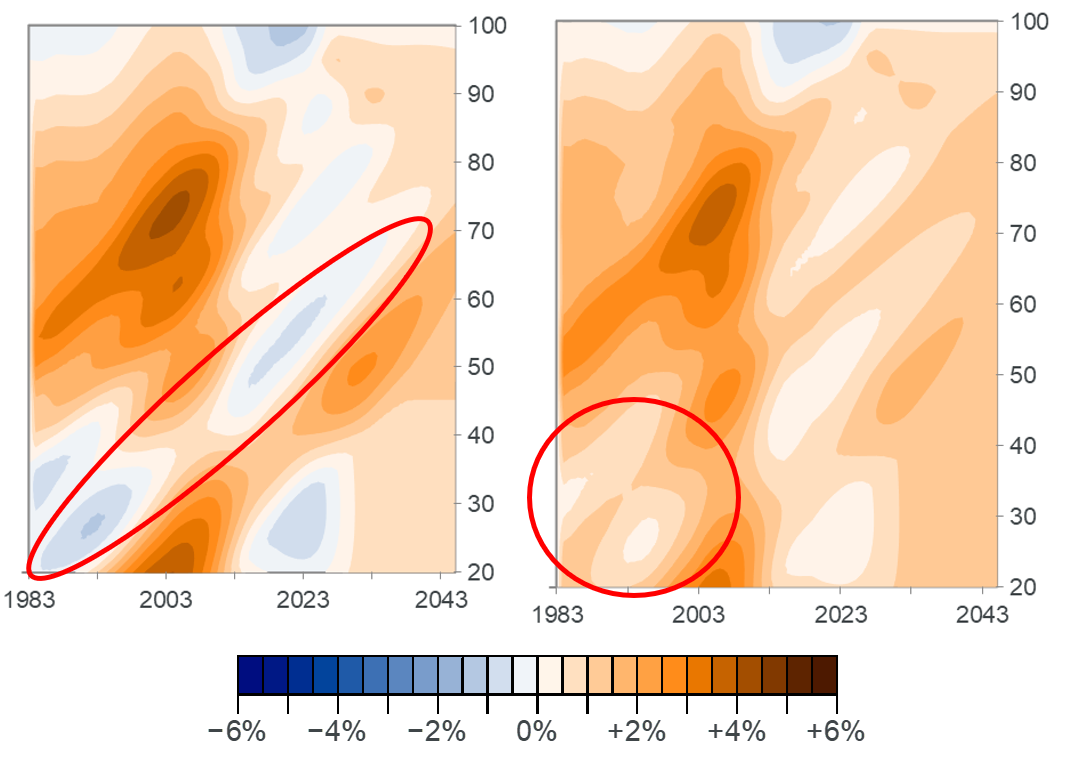
Source: Default CMI_2023 model applied to England and Wales population data from the Office for National Statistics, as processed by the CMI
The heatmap above shows the model output by age and calendar year for a recent version of the CMI model (CMI_2023) using the default model calibration. Warmer colors represent higher positive improvements and cooler colors depict lower or negative improvements. Cohort effects appear as diagonal patterns in this type of chart because the effect is assumed to stay with specific people as they age (moving upwards) over time (moving left to right). Whether the projected patterns produced by the model are sensible is explored further below.