“Predictive Modelling involves a process by which current or historical facts are used to create predictions about future events or behaviours.”
– Tim Rozar, Vice President, Head of Global Research and Development, and Scott Rushing, Vice President and Actuary, Global Research and Development, RGA Reinsurance Company
Introduction – assessing predictive models
Over the past few years, the concept of predictive modelling (PM) has entered the lexicon of many life insurance underwriting departments. For some, the basic concepts of multivariate regression analysis, generalized linear models, or specific methodologies such as the Cox proportional hazard model might be old news; for the majority it is likely a new area requiring development of a new set of skills or training to fully understand, assess and implement.
Years ago, underwriting saw a game-changing series of events, the HIV epidemic, followed by increased blood chemistry ordering, out of which the concept of preferred risk classification saw fruition. Predictive modeling is a powerful tool; does it have the potential be a game changer of the same magnitude? If so, should we expect the unexpected?
If we are going to play a statistical game with life insurance, we need to understand how PM fits as a game piece. Where does modeling fit as a business tool? How do the development, assessment and implementation affect the business? What does the company stand to gain, and are there risks? If the risks exist, how do we mitigate them?
Where should we apply predictive modeling resources?
- Lowering price
- Lowering underwriting costs
- Improving placement
- Reducing lapsation
- Fraud detection
- Efficient marketing
- Targeting attending physician’s statements
What is the proposed model supposed to predict?
- A client’s buying behavior
- A client’s response to a direct marketing campaign
- The underwriting department’s determination of preferred
- The increased mortality of a subset of clients
- A laboratory outcome
- The likelihood the client, agent or some other entity is committing fraud
- Identifying opportunities for cross selling
- That taking particular medications are predictive of higher than expected mortality
Who developed the model, and who comprised the team, including the mathematical work and the knowledge experts?
- Actuaries or statisticians
- Physicians
- Laboratory experts
- Underwriters
- IT and data professionals
To what purpose was the model developed? Where is the benefit?
- Reduced cost
- Business efficiencies
- Higher placement
- Improved overall mortality
- Better differentiation of the insured population
- Fewer lapses
- Better response rates on a direct marketing campaign
Modeling in life Insurance applies to all of the above in time. U.S. life insurance companies may vary in their current focus, but every day more companies are developing plans to integrate predictive modeling into their business. The business focus is on how to implement models that make business sense and improve overall mortality, or bring business that is more profitable. As an insurance professional, I know that the techniques have far wider application and it is important to know where and how the techniques are used and how effective they may be.
Let us spend some time discussing some of the issues to resolve in assessing the usefulness and the business case for implementing a predictive model into your company’s business practices. We can start by discussing modeling’s relevance, risks, goals and motivations, and move to a brief discussion of development, assessment and implementation.
Predictive modelling is relevant
Patterns may appear in data that are too subtle to appear in small samples. Data is the critical first component of any model. The use of data-mining techniques allows us to analyse data and plot the data in such a way that we can see patterns not obvious when looking at single variables.
Interactions between variables can become more apparent. The variables, and synthetic variables derived from them, can exhibit subtle changes often only seen, observed, and analysed in larger portions, from a multivariate approach.
Models can be more consistent than humans can. Once a model is developed, the variables properly weighted, and the algorithm established, and less prone to human lapses in attention, it should generate consistent results.
Properly implemented models can provide efficiencies and cost savings. Resources are always limited. When models optimize limited resources, the company should gain from those efficiencies. We still must be alert to the risks, and never forget there can be unintended consequences with any new business process.
Predictive modelling use must be cautionary
Models may be consistently right or (sadly) wrong. Predictive models can be a powerful business tool, but like any tool can be misused, misunderstood or
misinterpreted. If the developers do not understand the various elements in the same way the knowledge experts do, there is a risk the numbers (data) take on a life of their own, and fail to represent reality over time. Models are not reality – they are a representation or a surrogate. They can be good representations or bad abstracts. After all, model scores do not tell us facts, they provide us some form of weighted statistical probability that a given situation exists.
So as a way to apply statistical methodologies, or in a sense to influence a ‘bet’, predictive models also fall under some of the same rules of game theory that other economic predictors do. We will discuss this a bit later.
Predictive models are not ends in themselves, unless you are selling them. Therefore, there are two points to consider here. Going back to the idea of goals and motivations, the model is not the goal. The goals more likely revolve around efficiency, cost savings, or profitability. The second point is that, if you sell a model, it had better meet the client’s goals in an understandable way.
Making the business case – establishing the motivation, framing the problem, setting goals
Understand what the model purports to do. Models are surrogates, they are representations based on mathematics and statistical manipulation of data. Underwriters must remember that we underwrite proposed insureds, not scores.
When making a business case regarding the use of predictive modelling, spend most of the process identifying the symptoms or problems you are trying to solve. The time spent identifying the goals and objectives will provide the development team clarity and purpose. Without clear objectives, the model may end up solving the wrong problem.
The predictive model should present a solution to your problem from a particular frame of reference. Predictive models are a tool representing a data-driven and statistical frame of reference. Underwriters, medical directors and actuaries are no strangers to that point of view. That group also understands that models and averages, such as the law of large numbers, do not do a particularly good job at the individual level.
We can argue that using predictive modelling is not too much different from any other new business application. When assessing a newly developed laboratory test, or assessing a new underwriting requirement brought to us by a vendor, we need to perform adequate due diligence before we proceed with a long-term plan.
Development or Application of a model
Build it or buy it; we must first understand it.
Not unlike a software development initiative, or a life insurance company’s decision to use an actuarial consulting firm for product development, we have to determine whether we should build or buy. Does the company have the native expertise to analyse the data and develop the model? Does the company have sufficient expertise with the tools of predictive modelling, SAS or R for example? Should the company build the expertise or bring in experts already familiar with the techniques?
If you build it, you can control and monitor it. If you buy it, you must perform a different set of due diligence. You may own the data, but who owns the model?
Model development – a brief introduction
Before the work of modelling begins, the company must identify the goals and motivations for doing the predictive modelling work in the first place. Increase efficiency? Lower operating costs? Improve mortality? The modelling team will need knowledge experts to provide insights to those goals and motivations, as well as what the individual data elements and variables mean to the experts.
Models begin with data mining. The first diagram on the previous page provides a flowchart of the build process. The subsequent table is draw from several presentations by RGA’s Actuarial Research group. Together, they provide a bit of visual assistance on how the data feeds the build process, and the steps we need to complete a working model.
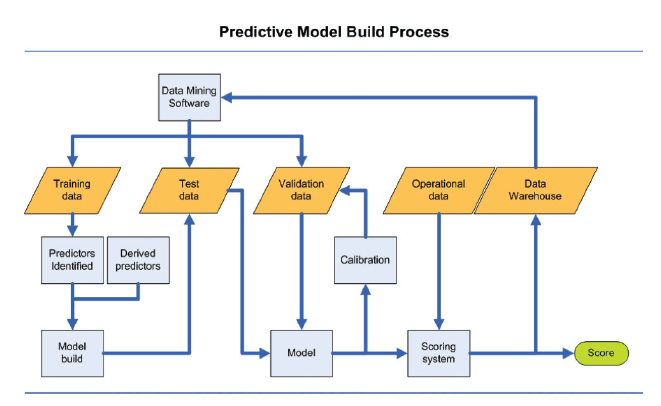
Modelling Process – another view
The following chart is drawn from various presentations by RGA’s Actuarial Research associates. It represents a slightly different presentation of the predictive modelling process.
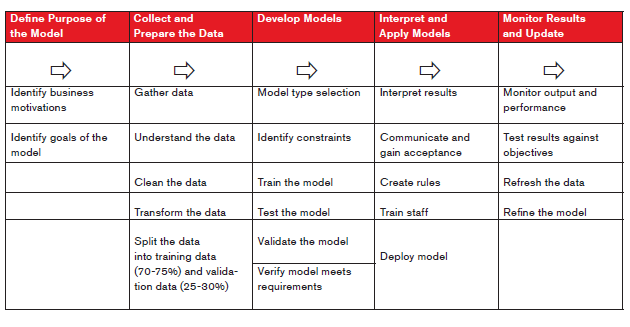
First, collect the data. Collecting data is something most companies have been doing for decades. We collect data, report management information, and watch trends. However, how often do we look for innovative ways to make the data do useful work? Predictive modelling uses past data, and the numerous variables and elements we mine from it to show where efforts are more effective, are less costly, stay on the books longer and show improved profitability results. However, the models only do that if developed using good technique.
All data requires cleaning and scrubbing. For all our good intentions, we still fail to have the structured data in a pristine format. Omissions, typographical errors, incomplete records, incorrect dates, and obviously inconsistent elements are all roadblocks to successful model development and implementation. We have to face the truth that most data sets need a thorough cleaning. Data-mining techniques require the data to be free of error as much as is possible. Once cleaned, the data will require structure in such a way that statistical analysis and statistical validation flow easily. Separate the data from the statistical noise. When plotted in various ways, patterns of behaviour may emerge. Draw data from the same source, at the same time, properly randomized, and divided, through the stages of model development:
- Assign the data distribution to two (at minimum) or three portions: training, test, validation
- Use training data to identify factors and predictors
- Establish the logic and algorithm
- Develop decision trees
- Model builds require some trial and error to achieve the most lift
- Build the model
- Build multiple models attempting to optimize lift (lift describes how directly the model predicts incremental impact on an individual behavior)
- Test the models using a portion of the identified data
- Watch for discordancy
- Calibrate
- Validate
- Scoring system established
- Implementation, use and maintaining a good model
- Establish audit procedures and feedback to data warehouse
- Calibrate again and frequently
Once a model moves to a production environment, the results require regular monitoring and recalibration.
Assessment
The assessment of a model goes beyond whether the model predicts accurately. As a business tool, we need to look at how the model fits into current practice and what change we anticipate after implementing the model. While changes might directly affect the model, they most certainly affect the business.
Bring the assessment resources together. The team should be multi-functional and include many of the company’s experts. Analyse the model through various frames of reference and points of view. Underwriters and medical directors, actuaries, marketers, sales, IT, and do not forget to include compliance participants. Application of the model will require a good explanation to all the participants, and also regulators.
Question the development team, to understand the model as completely as possible. Challenge the developers to explain the model in as few words as possible. Ask yourselves some tough questions, as you would with any new requirement or tool. What is the probability that the underwriter will identify a bad risk? What is the probability that the underwriter will identify a good risk as bad? Assess the technology necessary to feed the model. Study the economics, the cost benefit analysis and the protective value.
We also have to be sensitive to the credibility of the data. Terabytes of data can be subject to lack of credibility if the data cells are too finely divided or certain classes (variables) under study are infrequently encountered.
Questions to ask the developer or vendor
Now we turn our attention toward predictive models targeting life insurance risk. What are the questions we need to ask when confronted with a new predictive modelling proposal? What critical thinking skills and tools can come to bear on the assessment process? Sometimes we can start with a checklist. For example, RGA has developed a questionnaire to assist in the evaluation of predictive models. Those questions can guide discussions regardless of who develops the model under consideration, whether internal resources or a vendor.
The areas of questioning include:
- General questions regarding the purpose of the model and the techniques and data used for development
- Implementation
- Data and Variables
- Modelling Approach and Validation
- Maintenance
- Liability
- Future Plans
RGA would be happy to provide you with a copy of the questionnaire, if you contact us. A good checklist is a start, but there are other modes of assessment. Other statistical approaches and business strategic tools aid in the assessment. We can look at two, Bayesian and SWOT.
A Bayesian perspective
Most readers of ReFlections are familiar with the concepts of prevalence, exclusivity, sensitivity and specificity. We would be remiss if we fail to ask ourselves whether the modelling method used can deal with these important concepts. Let us assume the predictive model in question provides a score that we can translate into a relative mortality. Given that models are by their nature developed from experience, we should be sensitive to the bias that comes with that. Using your own data, the model is looking at prior applicants and insured lives. Using an outside source or vendor, the model may have used general population data, their own tested data from multiple clients, or perhaps your company’s data. Regardless of the source, we should ask some questions:
- What is the prevalence of the issue studied in the data set used to develop the model?
- Can we assume that prevalence is the same in the future applicant pool?
- Do new marketing strategies that seek new sources of potential clients change the previous answer?
- How sensitive is the models outcome? Does it ‘catch’ all of the applicants?
- How specific is the outcome? Does it ‘catch’ only the appropriate applicants?
- What is the positive predictive value?
- What is the negative predictive value?
- How many in the potential applicant population are preferred, substandard, or even more significantly impaired?
- How much exclusivity can we assign to the model alone?
- Should we be running validation tests on applicants to confirm the score is consistent over time? In addition, what timeframe is reasonable?
Are we prepared to be wrong more often than we are right? As an example, in a test (PM score?) with 99% sensitivity, and 98% specificity, we would be wrong twice as often as we would be right. Imagine we have a test that identifies a disease with a prevalence of one in 1000 of the studied population, and the test is 99% sensitive and 98% specific. When we test 100,000 individuals, we get something like the following results:
- 990 true positives (have the disease and are correctly identified)
- 10 false negatives (have disease, but are missed by the test)
- 1,980 false positives (identified as having disease, but do not)
- 97,020 true negatives (do not have the disease and also test negative)
The number of falsely identified individuals is twice the number of accurately identified individuals. A clinician overcomes this problem by either repeating the test, or performing some other reflexive test. The labs also use reflexive strategies to limit the number of false positives. We need to consider whether we hold predictive model scoring to the same standard.
Of course, we must evaluate each predictive model on its own merits looking at the prevalence, sensitivity and specificity for the modelled population.
SWOT analysis – your analysis may vary
SWOT analysis involves reviewing strengths, weaknesses, opportunities and threats. As a business exercise, it provides a tool for seeking a balanced assessment of a new strategic plan. A company can use the tool to play to its strengths and develop mitigation strategies against its risks.
The following list is offered for consideration without implying comprehensiveness.
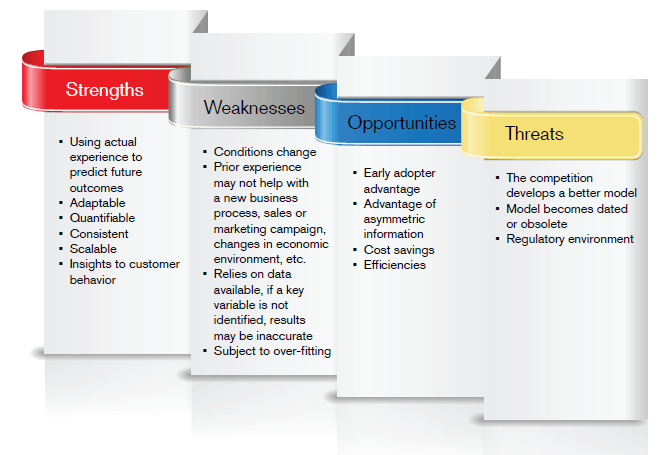
The goal of the SWOT analysis is to understand the good and the less-than-good aspects of modelling. While only one managerial approach to assessment, the SWOT can be a powerful tool albeit different than the tools underwriters and actuaries use more routinely – the cost-benefit analysis and the protective value study.
Perform the cost-benefit analysis and protective value study
The complementary tools of our industry, the cost-benefit analysis and protective value studies, have their place in this process as well. Does the cost of development and maintenance of the model provide more benefit than the traditional approach? How much protective value does the score provide exclusively? Theoretically, certain variables in a model would already be factors (right or wrong) in the underwriting process. How do we adjust the model’s value in those circumstances?
Do we have enough information to analyse either the cost-benefit or the protective value adequately? Can we determine the exclusivity of the ‘new’ information the model provides? To what degree does the model actually meet its stated goals?
Problems with modelling techniques
Many of the models currently under discussion in the life insurance industry are generalized linear models, such as the Cox Proportional Hazard model. They are well studied, and relatively easy to work with. GLM prevail in wide use because of their versatility and applicability. However, like any statistical tool they are not immune to flaws in implementation or design.
While we can summarize a wide variety of outcomes, we can experience problems. The developer / statistician/actuary is responsible for specifying the exact equation that best summarizes the data. If the GLM does not have the correct specifications, the estimates of the coefficients (the ß-values) are likely to be biased (i.e., wrong). The algorithm that results will not describe the data accurately. In complex scenarios, the model specification problem can be a serious one, difficult to avoid or correct without significant analysis and effort.
The problem of over-fitting
Quoting the omnipresent Wikipedia - The Free Encyclopedia:
“In statistics, overfitting occurs when a statistical model describes random error or noise instead of the underlying relationship. Overfitting generally occurs when a model is excessively complex, such as having too many degrees of freedom, in relation to the amount of data available. A model, which has been overfit, will generally have poor predictive performance, as it can exaggerate minor fluctuations in the data.”
One of the principle reasons to parse data into specific portions is to avoid this problem. Using all the data available to build the model might initially sound wonderful and it might be possible to describe perfectly the data with some algorithm. However, such models might become very complex as the developer tries to fit each record into the model. A wonderful way to describe what has been, but questionable on how accurately it will describe what will be.
Predictive modelling and the Theory of Games
Until the movie A Beautiful Mind was released in 2001, few people outside the economics profession had heard of game theory. When future Nobel Laureate John Nash, portrayed by Russell Crowe, was describing to his friends why it didn’t make sense for each of them to try to date the most beautiful girl in the bar he was describing a game theoretical approach toward obtaining a limited resource, in this case ‘dates’.
He showed that when every one of his friends pursued the most beautiful girl they would block each other and fail. They would then also fail with her friends. The only way to ‘win’ as a group was that they should ignore girl number one, and each pursue a different girl. Therefore, the game had a goal, and each of his friends wanted to win, yet by all seeking the exact same goal at best only one could win and in his suggested scenario, all of his friends get dates. This is a solution to the Nash Equilibrium problem. By the way, the solution in the movie is not the only solution, not even the only optimal solution. It did make for an interesting bar scene with a table full of math geeks.
In the movie Moneyball, predictive modelling and game theory provided a means to optimize players as a group rather than viewing them as individuals. By looking past traditional ways of ranking players, the Oakland A’s baseball team was able to develop a technical advantage, albeit relatively short-lived, in order to put together a winning team. Today many teams use the “Sabermetrics” analysis process to assess players, prospects and the team.
One of the principle goals of game theory is to optimize results, for the individual and the group. I propose that predictive modelling, if properly applied, can in fact optimize results for all parties involved; however, it takes work. We start that work by realizing that the company, by being efficient in its process, can and should bring some additional benefit to the other interested parties. Given enough time and analysis, is it possible to optimize underwriting by finding the best strategy for underwriting and acceptance of risk, declination of risk, underwriting cost, percentage of preferred issues, the optimal lapse rate, etc. to maximize the company’s profit? Probably not in these early days, but as we improve at these skills, who knows what will become possible?
While there are many players in the game of life insurance, let us limit our review to two – the company underwriter and the proposed insured. In our game, we have asymmetric information. The two parties know different things, and may not be privy to what the other ‘player’ knows. Generally, we argue the advantage goes to the proposed insureds who know more about themselves, their medical history, and their life styles than we do. We combat that advantage with sentinels, MIB, examinations, blood chemistry, urinalysis and pharmacy record checks. In a few cases, we have access to information the client does not know or has forgotten. After all, many physicians do not open their charts to their patients.
If companies begin using predictive modelling to uncover unrecognized factors or variables unknown to the client, they shift the asymmetric information equation a bit their way. Fair enough, we should be able to shift the balance assuming no intervention from regulators or compliance if the equation suddenly shifts in the company’s Favor.
From another viewpoint, if the model actually improves the client’s price or opportunity to buy, we might optimize the game so that everyone wins. “Everybody wins” is usually a good long-term strategy from an economic standpoint.
There is a possible downside to using models, whether complicated or not. The sentinel effect whereby the client’s knowledge that the company is screening for some factor will cause certain people to take their business elsewhere may not be as helpful in a predictive modelling environment. Would the average applicant understand the complexity of a model?
The topic of integrating a model in light of game theory could actually extend this discussion for some time, and some will return to it another day.
Summary
Predictive modelling is here to stay. In the future, we will see models in a realistic light and with reasonable expectations of performance. While modelling will change the game in some ways, life insurance must still be properly priced and underwritten. Modelling is a new game piece, and as in chess, all the game pieces have their individual strengths and weaknesses. The pieces are strongest when applied in the correct way, and in combination to provide an optimal multi-faceted solution.
The scores and outcomes of predictive models promise a new approach to this game we hope to improve. New tools provide new insights, and new opportunities, many of which we cannot foresee. It should be an exciting time. As we begin the application of predictive model scores, critical thinking, assessment and preparation by all involved will guide success with the new rules of the game.
I will close with one thought that should be foremost in our minds and close to our hearts, lest we forget: To paraphrase Dr. Holowaty, “We underwrite individuals, not predictive model scores.”