"All models are wrong, but some are useful." This aphorism from statistician George Box is important to keep in mind because it seems that wrongness is often used to dismiss modeling efforts as useless.
Models are, by nature, approximations of real-world processes, and therefore they cannot be exactly right. Models can be useful if they approximate the causal mechanism and/or estimate the outcome of a process. Those two uses are not mutually exclusive, but different models tend to emphasize one or the other. Mechanistic models help us understand how a process works, usually with the goal of manipulating the process to some desired effect. On the other hand, predictive models allow us to plan for an anticipated outcome.
Both approaches have been used by various teams to model the COVID-19 outbreak. Mechanistic models by scientists at Imperial College London explored the influence of interventions on the trajectory of the outbreak. The early models from the Institute for Health Metrics and Evaluation (IHME) used a statistical approach to predict outcomes, including the resource allocation needed for hospitals to care for critically ill patients. This non-mechanistic approach was simultaneously their advertised “feature” and widely criticized “bug.”
The most common method for modeling a disease outbreak is the compartmental model. This is a mechanistic model that uses differential equations to describe the proportions of individuals that are susceptible, infected, or removed in a population at any given time.
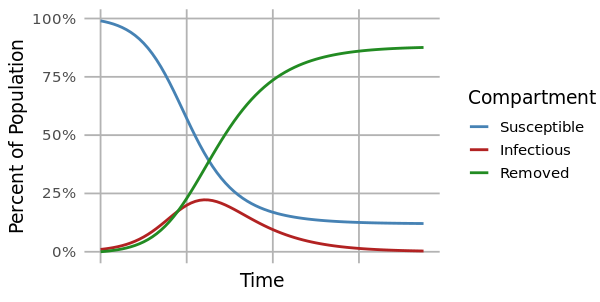
At the start of an outbreak, individuals move from the susceptible to infected compartments at a rate determined by the reproductive number (R0). This is the average number of secondary infections caused by an infected individual at the beginning of an outbreak (time=0), and it is related to the ratio of susceptible to infected individuals. As an outbreak progresses, that ratio changes, and the reproductive number at time=t (Rt) drops. When Rt<1, the outbreak begins to slow.
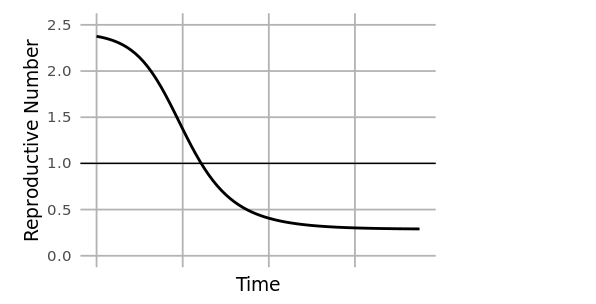
A limitation of the compartmental modeling approach is that assumptions of uniformity must be made about the population being modeled for a single set of model parameters to represent the entire population (uniform population density, uniformly random mixing, uniform probability of transmission, etc.). In reality, substantial variation in things like population density from urban to rural settings, and differences in containment policies from jurisdiction to jurisdiction might mean that parameters like Rt differ among subpopulations. Under these conditions, a single population model can be “more wrong” and “less useful.” To overcome that problem, a meta-population model may be used to first model individual states or cities, and then aggregate those results to a national level.
Individual-based models (IBMs) like the Imperial College model take this idea further and model the behavior of each individual of a population. IBMs are computationally intensive, but a powerful tool for discovering emergent patterns from highly heterogeneous populations.
Many COVID-19 models have been made freely available to the public. The Reich Lab from University of Massachusetts - Amherst has taken projections of more than a dozen of the leading published models (including Imperial College and IHME) and created a four-week ensemble prediction that updates itself as new projections become available. Harvard produced a model that examines various scenarios of seasonality, immunity, and containment policy to project transmission dynamics of the virus over the next five years. While a five-year projection is almost certainly "wrong," it is useful to examine the range of different scenarios possible.
Summary
Dozens of models have been developed by researchers around the world to study the COVID-19 pandemic. All of them are wrong but many are useful to help us understand the spread of the disease and the range of possible outcomes.