Accelerated underwriting (AU), a fully underwritten process in which some requirements are waived for a portion of applicants demonstrating favorable risk characteristics, has
swept the industry at an incredible pace in recent years.
Virtually nonexistent five years ago, AU is offered by more than 45 U.S. life carriers today, with still more on the horizon. For many of these carriers, the most pressing questions have now shifted in theme from the development and launch of an AU program to strategies for effective monitoring and management of an AU program.
The longevity of the prior fully underwritten paradigm has given us a much clearer picture of how key assumptions will be impacted when a new product – or even a slight underwriting change – is introduced, a direct result of 20-plus years of credible experience. Accelerated underwriting is so new, there is no credible experience available yet, and adjustments may be needed when using fully underwritten experience to inform development of assumptions for an AU program. Adding to the challenge, many of these adjustments by their very nature cannot be estimated from historical analysis, and those that can may manifest at different levels in production.
This uncertainty underscores the risk of letting an accelerated underwriting program run on autopilot. Any AU program will, at a minimum, require monitoring to gauge performance against initial expectations. It may also need to be refined and updated over time. Monitoring results may also be requested by reinsurers and regulators who consider its impact to reinsurance pricing and the appropriateness of valuation assumptions under principle-based reserves (PER). This level of program management is achieved only by analyzing data captured from real production cases that have been processed by the AU program.
Collecting the Data
Monitoring is important for any AU program, but exactly what kind of data should be collected, and how is this accomplished?
At a basic level, it is beneficial to store all evidence used in making an accelerated decision, including third-party evidence and, to the extent possible, application disclosures. Beyond that, most AU-monitoring programs use some combination of pre- and post-issue auditing. Pre-issue audits are most commonly performed through random holdouts in which all age and amount requirements are ordered and full underwriting is applied to a certain percentage of cases that would ordinarily qualify for an accelerated offer. Post-issue monitoring involves ordering additional evidence after the policy has been placed in order to check for undisclosed information. Attending physician statements (APS), post-issue prescription histories, and MIE Plan F are commonly used here.
Both pre- and post-issue audits can be beneficial, but they should not be viewed as interchangeable. Pre-issue audits provide the truest comparison between results from the new AU program and the prior fully underwritten process, as all of the
necessary evidence is available to produce both an AU and a fully underwritten risk class determination. This apples-to-apples comparison isn't possible with post-issue auditing, because the underwriter will not have access to all of the evidence that would have been used in full underwriting. A post-issue APS, for example, will almost always be missing key lab tests (e.g., a cotinine test) performed as part of an insurance lab panel, if it has blood work at all. Pre-issue audits also have the secondary benefit of catching and removing misrepresentation before policies are issued for audited cases.
Post-issue audits are performed on policies that have already been issued through the AU process. Therefore, deliberate action, including after-issue rate class adjustments and possibly even live rescissions, is required when material misrepresentation
is discovered if the quality of the business is to be impacted. However, post-issue audits may uncover types of targeted misrepresentation that could be missed through pre-issue auditing. For example, if an applicant is aware of a medical risk factor but avoids consulting a physician until after applying for life insurance, evidence of this condition may be absent at the time of initial underwriting. Cases like this could possibly be discovered through tools such as a new post-issue prescription history check.
Many carriers have found a combination of pre- and post-issue audits to be the best approach.
Whatever tools and methods are used to audit the AU program, it is critical to capture information on both the class that would have been offered through the AU program and the class that would have been offered based on the additional information discovered through the audit process. In cases where these two are different, the information leading the underwriter to make a different decision with full evidence should also be captured.
Accelerated cases should also be distinguishable from cases for which full requirements are still ordered so that these two pieces of the business can be studied independently as experience emerges. This should make its way into administration reporting
for reinsurers as well so that any downstream reporting can appropriately reflect the differences between these two groups.
Using the Data
Once the data are captured, it is possible to start evaluating the AU program performance against initial targets and expectations. The key performance indicators (KPls) used to quantify the main program goals at launch will often guide the focus of these efforts. Goals are commonly developed for acceleration rate (what percentage of applicants receive an accelerated offer) and mortality slippage (how much mortality changes relative to the prior fully underwritten baseline), along with others related to expense reduction, increase in placement rate and more.
With any of these metrics, it is first critical to understand the basis for the calculation.
When calculating the acceleration rate, the numerator is generally very clear: the number of applicants who received an offer without fluid testing, paramedical exam and/or other necessary tests. The denominator, however, can vary significantly from one carrier to the next. It could represent all applicants within the age and face amount limits of the AU program, or it might be limited to applicants who use a particular process (e.g., tele-app) or ones who first pass a list of pre-screen questions and criteria. Whatever combination of restrictions apply, it is imperative to be consistent between the definition used when setting goals for the program and the calculation used when analyzing production results.
Many carriers use a confusion matrix to estimate mortality slippage by comparing the prior fully underwritten class of an applicant to the class that person would receive under the new AU program. A live confusion matrix (see Figure I) can be populated based on results from random holdouts in order to evaluate the program's performance in production. In this example, the level of accurate classification is mostly as expected in the top two classes. However, more tobacco cases than expected slip through to an accelerated non-tobacco class. This technique can also be used with post-issue audits, in which case it will also be important to consider the impact of information available from prior evidence (generally an insurance lab panel and a paramedical exam) that may not exist in the evidence used in the audit (e.g., an APS). Once again, the more consistent the confusion matrix is with the targets developed when the program was designed, the more valuable it will be-a principle that applies for all of the program's KPIs. Taken together, this analysis shows whether or not the program is meeting its targets and the magnitude of any discrepancies.
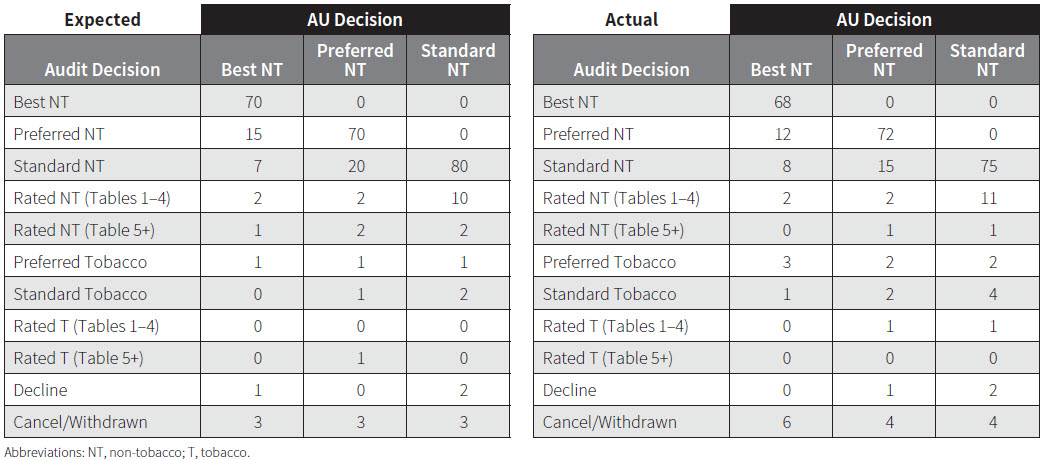
If variances exist from expectations (as in Figure 1), other metrics can be useful in determining the causes for that deviation, answering the questions "How?" and "Why?"
Applicant (and, in some cases, agent) behavior is a major unknown when introducing an AU program. How will applicant disclosure change in the absence of known testing? Will the change in process encourage agents to engage a materially different group of risks than they have in the past, or could the introduction of the AU program attract new applicants? Comparing distributions by demographic variables (age, gender,
risk class, etc.) and third-party evidence pre- and post-AU can illustrate whether the applicant pool is changing. Analyzing misrepresentation for verifiable risk factors can show how applicant behavior is changing.
In Figure 2, tobacco nondisclosure seems to be tracking at a 50 percent increase relative to the pre-AU baseline. Similar analysis can be performed for other risk factors, such as build (Figure 3). Results like this can help identify the root causes for variance from expectations uncovered in the confusion matrix.
Figure 2:
Random Holdouts, Tobacco Nondisclosure
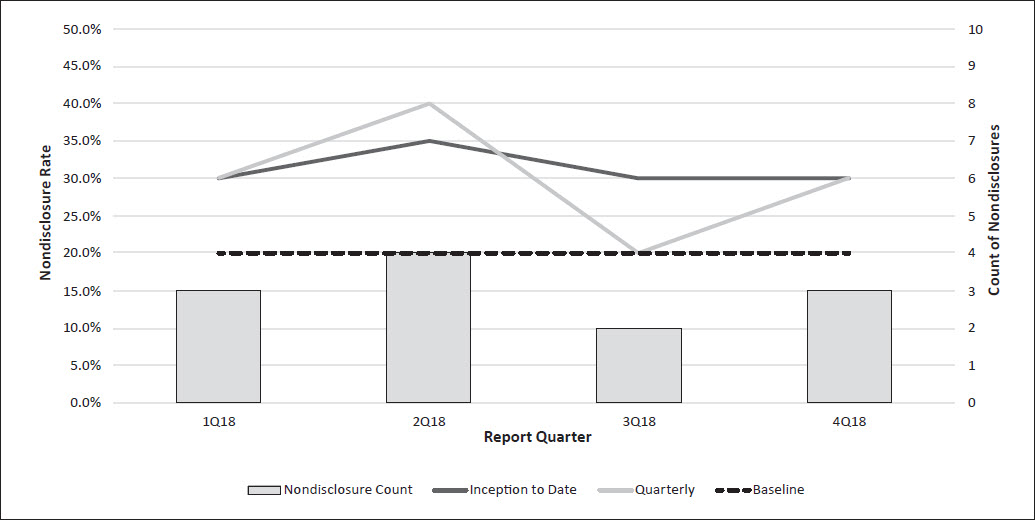
Analysis of more traditional measures can be useful as well. It will likely take some time for credible mortality experience to emerge, but analyzing causes of death on early claims (particularly for accelerated cases) can help identify possible holes in the AU process. Tracking distributions by risk class can show if the shifts projected at program launch are materializing. For example, if more individuals than expected are offered Super Preferred, this could suggest that nondisclosure is higher than expected or that certain application questions or rules allow more cases than expected to slip through the cracks. Similarly, face amount and tobacco disclosure trends by agent, such as an agent suddenly selling many more cases at the maximum AU face amount or an agent with no admitted smokers, can indicate areas for further investigation.
Even the rate at which applications are withdrawn-particularly at certain points in the process-can provide useful information. Although somewhat informative on its own, this type of data is most useful when paired with pre-AU values to provide context. Referring back to Figure 1, the withdrawal rate is higher than expected for the best class. If these applicants withdrew when additional testing was required, that could be an indication of possible anti-selective behavior. Similarly, in Figure 2, the level of tobacco nondisclosure would be less of a concern if the prior baseline were 30 percent, as that would seem to indicate applicant behavior is not changing in the absence of testing, and this would have been reflected in the initial assumptions.
Conclusion
As more carriers move past the launch of their accelerated underwriting programs, the ongoing management of those programs will start to become an area of much greater focus. A robust monitoring program will be at the core of these efforts for many carriers. This will provide leading indicators of program performance and will also highlight areas for potential improvements (e.g., revised application wording and adjustments to score cut points), both of which will be crucial to sustainable success. This becomes more critical in a PER world as carriers seek to justify their mortality assumptions in the absence of a credible experience study for their AU programs.
It is crucial that carriers check the gauges on their AU programs frequently in order to both capitalize on the opportunities presented by accelerated underwriting and steer clear of obstacles in the road.